Should you Build or Buy your AI?
As AI technologies have matured and the ecosystem of reliable vendors has expanded, the decision to build vs. buy AI is no longer theoretical - it’s strategic. The early days of bespoke AI systems built from scratch are giving way to a more nuanced marketplace with reusable components, APIs, and managed services that can accelerate value delivery. With this maturity comes a new responsibility for technical leaders: to choose where to invest in proprietary capabilities and where to lean on external platforms.
Lets take a look at how we can evaluate if our AI use-case should be bought or build from scratch in-house.
Build vs. Buy Decision Matrix
This 2x2 matrix evaluates opportunities based on the following:
Y-axis - Strategic Value of the Data: Is the data a source of differentiation or merely operational?
X-axis - Vendor Access to Similar Data: Does the vendor have equivalent or superior datasets that fuel their model performance?
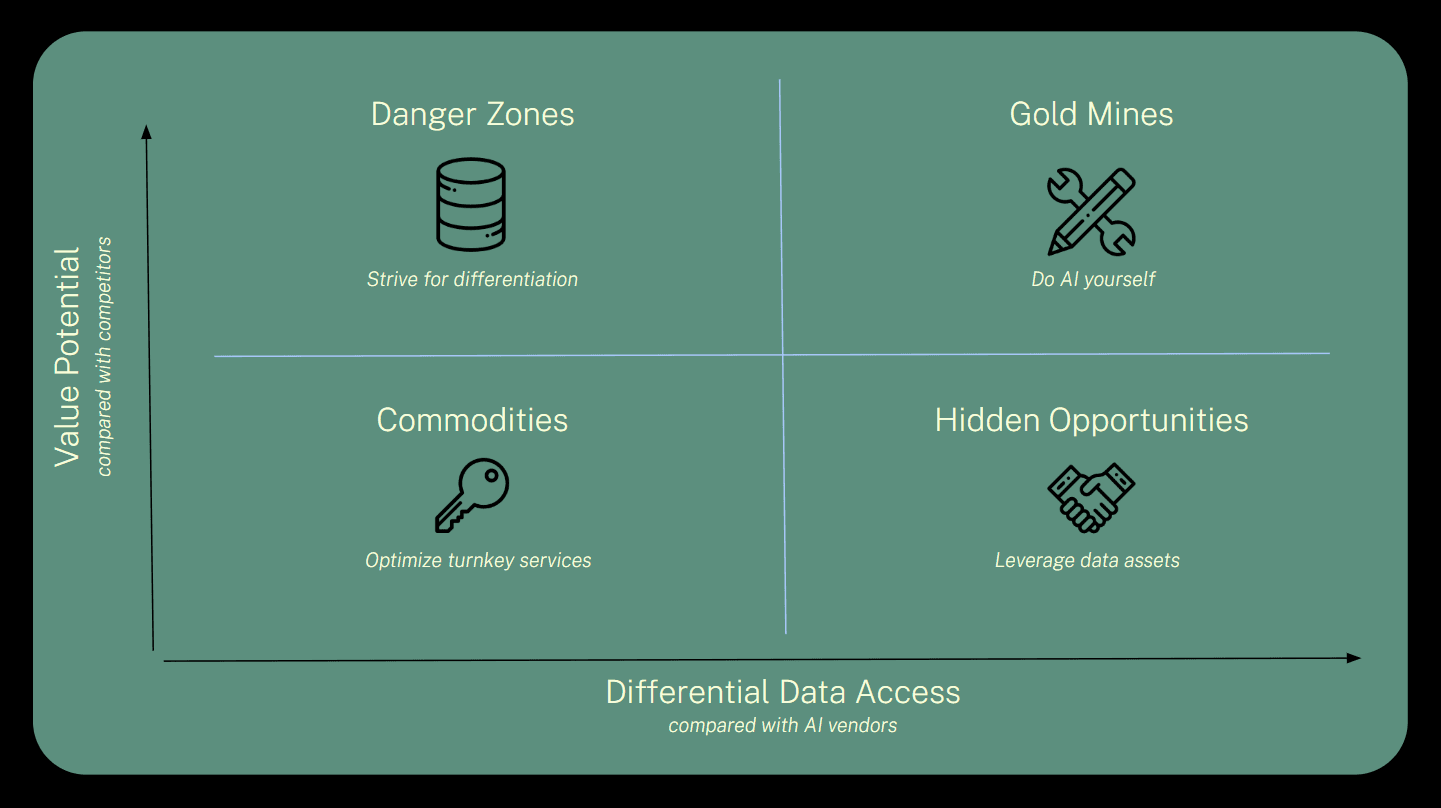
By plotting use cases against these axes, we uncover four archetypal zones: Commodities, Hidden Opportunities, Danger Zones, and Gold Mines.
Commodities
Anything in this area should replace repetitive back-office effort with off-the-shelf AI.
These domains involve standardized processes and abundant training data shared across organizations, such as HR forms, payroll entries, and financial records. The data is non-differentiated and operational in nature—it's critical for business continuity, but offers little to no strategic edge.
Vendors in this space have robust models pre-trained on a vast collection and can offer competitive SLAs, model performance, and integration timelines. Your organization's data doesn’t meaningfully improve model outcomes, and custom training typically provides diminishing returns. Because these use cases are well understood and widely applicable, off-the-shelf products can deliver 80–90% of the value at a fraction of the effort. The cost of building your own solution would be difficult to justify, both in opportunity cost and in long-term support.
Focus your internal teams on integrating these tools into your broader workflows, automating downstream processes, and ensuring strong governance and compliance rather than modeling from scratch. Ultimately, buy off-the-shelf AI in these areas as there is no strategic value in reinventing the wheel.
Hidden Opportunities
The key is to partner if working in this quadrant to customize or co-develop where off-the-shelf lacks specificity.
Here, your data is distinctive due to proprietary workflows, instrumentation, or legacy systems, but it doesn’t represent a core strategic differentiator. The uniqueness arises from operational quirks or industry-specific processes, not from innovation or competitive insight. Off-the-shelf models often fall short in these scenarios because vendors have no comparable data to fine-tune against, making general-purpose models underperform. This opens the door for co-development: your data improves vendor models, and in return, you gain optimized performance without bearing the full burden of R&D.
These situations are ripe for API-level integration with configuration layers, or joint ventures where domain expertise and annotated datasets are shared securely. Look for vendors that offer "bring your own data" (BYOD) models, private cloud deployments, or retraining options. It’s critical to establish governance models that define IP ownership, data residency, and retraining rights. While you’re not building the model yourself, you are helping shape it—and you should own the part that makes it better.
Engage in strategic partnerships with vendors. Customize where needed, but don’t over-engineer, instead focus on shared value without taking on unnecessary build burden.
Danger Zones
For use cases in this area, you need to make sure to avoid or carefully control, as you don’t want to outsource your differentiator.
In this quadrant, you're facing a strategic trap: vendors have aggregated better data than any single enterprise, often by servicing many clients in your industry. While their models may seem tempting due to high performance, they represent a form of knowledge monopoly you cannot match. The risk lies in offloading core business logic or customer insight into opaque APIs. You become a downstream consumer of decisions made by a system trained on cross-organizational data—and worse, your contributions improve that model for competitors too.
For highly regulated or high-value industries (e.g., finance, healthcare), you also encounter issues with data portability, explainability, and privacy. A vendor may comply with regulations, but that doesn't mean you're insulated from risk if your differentiating signal becomes just another datapoint in their platform.
If you engage vendors here, do so with strong data protections, clear boundaries around model reuse, and contractual safeguards for model IP. Otherwise, build your own—control over data, architecture, and continuous learning will become your only moat. Proceed with extreme caution or build in-house. Avoid vendor lock-in where AI decisions affect your core business differentiation.
Gold Mines
Here you must Build and Defend. Your data is your edge, therefore you need to invest in proprietary AI.
In this zone, you are sitting on truly strategic, differentiated data—be it behavioral telemetry, proprietary research, or network activity that no other company has at your scale or resolution. This data is your competitive advantage. Vendor offerings are often generic or underpowered here because they lack access to your type of data, and their models are not designed for your unique context. Outsourcing these use cases would not only dilute your edge—it would risk leaking signal to the broader market.
Investing in bespoke AI for these domains means owning your models, pipelines, and feedback loops end-to-end. This allows for continuous improvement, tight coupling with internal systems, and fast iteration on new business opportunities.
These efforts require mature MLOps practices, strong data engineering, and a commitment to long-term model evolution, however the upside is significant. The more data you accumulate, the smarter your models get, and the harder it becomes for competitors to replicate your success. Ultimately, Build proprietary AI here. This is your strategic territory so you own the models, own the infrastructure, and keep the learning loop internal.
Technical Considerations Across All Quadrants
Beyond the strategic lens, several technical factors impact the viability and risk of each option:
1. Data Infrastructure & MLOps Readiness
Building is only viable if your organization has robust data pipelines, version control, and continuous training capabilities. Without these, even strategically critical AI investments may flounder.
2. Privacy, Compliance, and Regulatory Complexity
Industries under HIPAA, GDPR, or FedRAMP may find themselves constrained even in the Hidden Opportunities zone. Emerging tools like federated learning, differential privacy, and confidential computing can help—but they require deep architectural consideration.
3. Team Composition and Internal Capability
AI isn’t just a data science problem—it requires cross-functional engineering, product alignment, and ongoing support. Buy when internal capabilities are thin; build when AI is a first-class competency.
4. Time to Value and Lifecycle Cost
Building may take longer, but buying can create long-term costs in lock-in, customization friction, and degraded model performance over time. Always model total cost of ownership (TCO), including retraining, cloud inference, and switching costs.
Every AI decision is a trade-off between speed, control, and strategic impact. That’s why a thoughtful build-vs-buy framework matters more now than ever. In some cases, buying accelerates delivery with minimal risk; in others, giving away too much control could erode your competitive position. This matrix provides a way to make those calls systematically: buy commodities, partner on context-specific needs, build your gold mines, and avoid dependency in danger zones. By approaching AI as a portfolio of investments rather than a single bet, you can maximize value while staying aligned with long-term business goals. The real advantage isn’t in the technology, it’s in making smarter decisions with it.